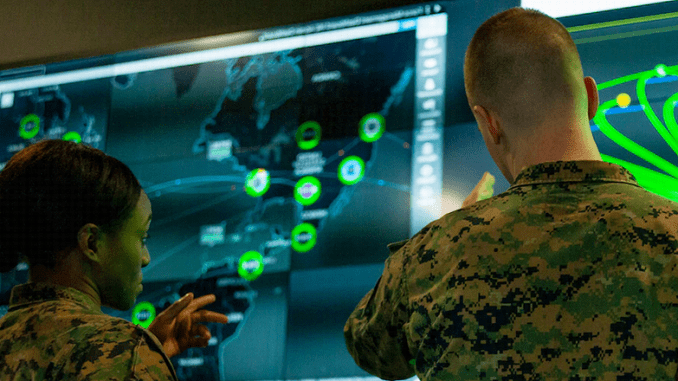
WASHINGTON, DC, October 4, 2021 (ENS) – A new international project is creating advanced artificial intelligence, AI, programs that will enable machines to learn progressively over a lifetime and share those experiences with each other.
Uses of this new technology could include co-operating self-learning autonomous vehicles such as self-driving cars, robotic rescue and exploration systems, distributed monitoring systems to detect emergencies, or cybersecurity systems of agents that monitor large networks.
Researchers hope the technology will allow machines to reuse information, adapt quickly to new conditions and collaborate by sharing information.
The project is part of the initiative Shared-Experience Lifelong Learning, or ShELL, a program funded by the Defense Advanced Research Projects Agency, DARPA. This U.S. government military agency is credited with some of the biggest technological advances in recent history such as the Internet, the miniaturization of GPS, Siri, and the computer mouse.
The project will be led by Dr. Andrea Soltoggio of the Computer Science department at Loughborough University, a public research university in the market town of Loughborough, Leicestershire, in England’s East Midlands.
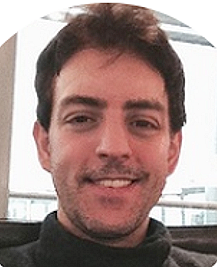
Dr. Soltoggio will partner with Dr. Soheil Kolouri at Vanderbilt University in Nashville, Tennessee, and Dr. Cong Liu at the University of Texas at Dallas.
“The idea behind this project is to gain a deep understanding of how and what an AI system learns when dealing with a new task, so that we can exploit task similarities and share information to create fast, reliable and collaborating learning agents,” Dr. Soltoggio said.
“One exciting aspect that goes beyond pure technological advances is that this research addresses high-level questions,” he said. For instance, “How can individual entities share information and benefit from each other’s experiences when learning together?”
“If one agent makes mistakes while learning a task, can this experience be shared with other agents so that they don’t make the same mistakes?” is another of the questions under exploration.
“Currently, these questions are mostly unanswered, but our proposal sets out lines of investigation to create such learning processes within AI agents,” Soltoggio said.
Each university involved in the project will build upon different aspects of lifelong learning, a new area of machine learning research that has grown also with the contribution of the previous Lifelong Learning Machines (L2M) DARPA program.
Loughborough will focus on “novel bio-inspired neural networks that learn shareable knowledge exploiting neuromodulation and synaptic consolidation mechanisms,” the scientists said.
Professor Claudia Eberlein, dean of Loughborough’s School of Science, said, “This funding from DARPA represents a phenomenal success for the university. It recognizes the international standing of our AI experts and Dr. Soltoggio’s position among the leading authorities in the world on AI.”
Vanderbilt University will concentrate on the algorithmic theory and statistical foundation of the learning mechanisms, and Texas University will focus on the hardware integration and deployment for potential transition to industrial and other real-world applications.
Today, when companies deploy artificial intelligence programs, they are likely using machine learning, a subfield of artificial intelligence that gives computers the ability to learn without explicitly being programmed.
“In just the last five or 10 years, machine learning has become a critical way, arguably the most important way, most parts of AI are done,” said professor Thomas Malone at the Massachusetts Institute of Technology Sloan School of Management, who is also the founding director of the MIT Center for Collective Intelligence. “So that’s why some people use the terms AI and machine learning almost as synonymous … most of the current advances in AI have involved machine learning.”
A 2020 Deloitte survey found that 67 percent of companies are using machine learning, and 97 percent are using or planning to use it in the next year.
From manufacturing to retail and banking to bakeries, even legacy companies are using machine learning to unlock new value or boost efficiency. “Machine learning is changing, or will change, every industry, and leaders need to understand the basic principles, the potential, and the limitations,” said MIT computer science professor Aleksander Madry, director of the MIT Center for Deployable Machine Learning.
While not everyone needs to know the technical details, they should understand what the technology does and what it can and cannot do, Madry added. “I don’t think anyone can afford not to be aware of what’s happening.”
Funding for the international machine learning project at Loughborough, Vanderbilt and University of Texas is part of DARPA’s Artificial Intelligence Exploration, AIE, program. In this series of high-risk, high payoff projects, researchers work to establish the feasibility of new AI concepts within 18 months of their funding award.
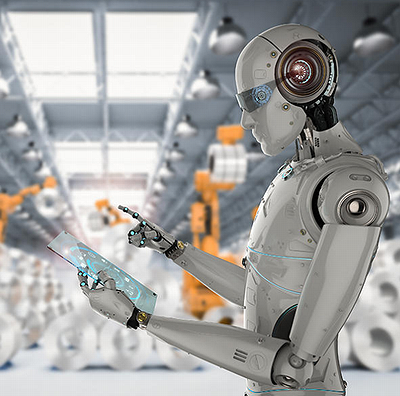
DARPA announced in September 2018 a multi-year investment of more than US$2 billion in new and existing programs. Key areas of the campaign include:
- – automating critical DoD business processes, such as security clearance vetting or accrediting software systems for operational deployment;
- – improving the robustness and reliability of AI systems;
- – enhancing the security and resiliency of machine learning and AI technologies;
- – reducing power, data, and performance inefficiencies;
- – pioneering the next generation of AI algorithms and applications, such as “explainability” and common sense reasoning.
DARPA will create powerful capabilities for the U.S. Defense Department by attending to these areas:
- – New Capabilities: AI technologies are applied routinely to enable DARPA R&D projects, including more than 60 existing programs, such as the Electronic Resurgence Initiative, and other programs related to real-time analysis of sophisticated cyber attacks, detection of fraudulent imagery, construction of dynamic kill-chains for all-domain warfare, human language technologies, multi-modality automatic target recognition, biomedical advances, and control of prosthetic limbs.
DARPA will advance AI technologies to enable automation of critical Defense Department business processes. One such process is the lengthy accreditation of software systems prior to operational deployment. Automating this accreditation process with known AI and other technologies now appears possible.
At the same time, the failure modes of AI technologies are poorly understood. DARPA is working to address this shortfall, with focused R&D, both analytic and empirical.
- – Adversarial AI: The most powerful AI tool today is machine learning, ML. ML systems can be easily duped by changes to inputs that would never fool a human. The data used to train such systems can be corrupted. And, the software itself is vulnerable to cyber attack. These areas, and more, must be addressed at scale as more AI-enabled systems are deployed.
- – High Performance AI: Computer performance increases over the last decade have enabled the success of machine learning, in combination with large data sets, and software libraries. More performance at lower electrical power is essential to allow both data center and tactical deployments.
- – Next Generation AI: The machine learning algorithms that enable face recognition and self-driving vehicles were invented over 20 years ago. DARPA has taken the lead in pioneering research to develop the next generation of AI algorithms, which will transform computers from tools into problem-solving partners.
DARPA is researching AI-specific hardware designs, and the agency is also attacking the current inefficiency of machine learning, by researching methods to drastically reduce requirements for labeled training data.
DARPA research aims to enable AI systems to explain their actions, and to acquire and reason with commonsense knowledge.
Dr. Matt Turek of DARPA explains, “Machine common sense has long been a critical but missing component of Artificial Intelligence. Recent advances in machine learning have created new AI capabilities, but machine reasoning across these applications remains narrow and highly specialized. Current machine learning systems must be carefully trained or programmed for every situation.”
Featured image: U.S. military service members work on Artificial Intelligence applications at a DARPA facility. June 2021 (Photo courtesy U.S. Department of Defense)